In an increasingly digital world, algorithmic decision-making systems play a pivotal role in shaping our lives. From job applications and loan approvals to healthcare diagnostics and criminal justice, algorithms are now involved in critical decisions that affect individuals and society as a whole. While these algorithms promise efficiency and objectivity, they are not immune to bias, often reflecting the biases present in the data they are trained on and the humans who design them. In this blog post, we will delve into the complex issue of bias in algorithmic decision-making, exploring its origins, consequences, and potential solutions.
The Genesis of Bias
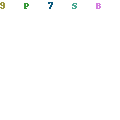
Bias in algorithmic decision-making often begins with the data used to train these systems. If historical data contains biases, those biases can be perpetuated and even amplified by algorithms. For instance, if a hiring algorithm is trained on a dataset that predominantly consists of male employees due to historical hiring practices, it may favor male candidates and perpetuate gender bias in hiring decisions.
Moreover, the designers of these algorithms can inadvertently introduce bias through their own choices and assumptions. The features selected for analysis, the weight given to certain factors, and the definition of success metrics can all influence the outcomes of algorithmic decisions. Inadvertently, or sometimes even intentionally, these design choices can encode societal biases into the algorithm.
The Ripple Effect of Bias
The consequences of bias in algorithmic decision-making are far-reaching and impactful. One immediate concern is the perpetuation of discrimination. Biased algorithms can unfairly disadvantage certain groups, leading to systemic discrimination in areas such as employment, housing, and financial services. This not only harms individuals but also perpetuates societal inequalities.
Furthermore, bias can erode trust in institutions and systems that rely on algorithms. When people believe that they are subject to unfair and biased decision-making processes, they lose confidence in the organizations using these algorithms. This can have serious implications for public trust in government, businesses, and other institutions.
The Quest for Fairness
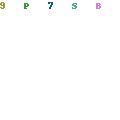
Addressing bias in algorithmic decision-making is a complex and ongoing challenge, but it is not insurmountable. Several approaches are being explored to mitigate bias and promote fairness in algorithmic systems:
1. Diverse and Representative Data
One of the fundamental steps in reducing bias is to use diverse and representative training data. By ensuring that datasets include a broad spectrum of characteristics and experiences, algorithms can learn to make decisions that are fairer and more equitable.
2. Transparent Algorithms
Algorithmic transparency is crucial for identifying and addressing bias. Making algorithms more interpretable allows for a better understanding of how decisions are made and helps to detect and rectify biased patterns.
3. Regular Auditing and Testing
Regular audits and testing of algorithms are essential to identify and rectify bias over time. Continuous monitoring can help in detecting and mitigating bias as it emerges in real-world scenarios.
4. Ethical Guidelines and Oversight
The development and use of algorithms should be guided by clear ethical principles. Regulatory bodies and oversight committees can play a vital role in ensuring that algorithms adhere to these principles and are held accountable for their decisions.
5. Inclusivity in Design
Diverse teams of designers and developers can bring different perspectives to the table and help mitigate bias at the design stage. This approach can lead to more inclusive algorithms that consider a broader range of perspectives and experiences.
Bias in algorithmic decision-making is a multifaceted issue that demands careful consideration and action. While algorithms offer tremendous potential for efficiency and objectivity, they must be designed and used with an unwavering commitment to fairness and equity. By addressing the origins of bias, understanding its consequences, and implementing proactive measures, we can work towards a future where algorithms empower, rather than discriminate, ensuring a more just and equitable society for all.
Leave a Reply